In today’s world, where data is as ubiquitous as the air we breathe, the ability to effectively distill value from the heterogeneous globs of information that underpin enterprises is of paramount importance across all industries. Knowledge modeling, the art of creating frameworks that define how data is comprehended at a human level, is not just a technical exercise but a strategic business initiative. This process serves as a bridge between the raw, typically-messy data generated by business activities and the actionable insights that inform more meaningful decisions.
This overlaying of business data objectives across the underlying data itself is fundamental in breaking down the silos that often isolate valuable information within separate systems or departments. By developing semantic models, or ontologies, organizations create a unified view that encapsulates not just the data but also its context and relationships. This holistic approach drives a more rapid generation of insights, accelerates decision-making processes, and enhances the overall agility of organizations by ensuring a broader, more meaningful comprehension of their data.
It is generally accepted that the creation of added value requires collaboration inside and between organizations. Collaboration requires sharing knowledge…
Rosing, Mark von & Laurier, Wim & Polovina, Simon. (2015). The Value of Ontology.
However, the complexity and scale of most organizations presents a daunting task. The ambition to “boil the ocean” by creating expansive ontologies that represent the ever-present nuances of a business’ operations oftentimes results in projects that become overextended and end up delivering no practical value. As in agile software engineering practices, we recommend a strategy of targeting smaller, “bite-sized” use cases to deliver immediate and incrementally scaling value. As in agile software process, there are process rails and patterns to effectively scale knowledge models as your efforts broaden. By narrowing the aperture of modeling efforts and demonstrating a practical return on investment, organizations can leverage these technologies for an oversized impact in their analytics and support meaningful insights across their missions.
Boiling the Ocean
The idiom ‘boiling the ocean’ succinctly captures the futility of attempting tasks that are too ambitious or unnecessarily complex. In the context of knowledge modeling and ontology development, this metaphor aptly describes the challenge faced by organizations attempting to exhaustively model every aspect of their operations and knowledge domains. The desire to create an all-encompassing semantic model often leads teams into a quagmire of philosophical discussions and theoretical perfectionism, where the pursuit of an ideal model overshadows the practical needs of the business. Don’t let the “Perfect” be the enemy of the “Good.”
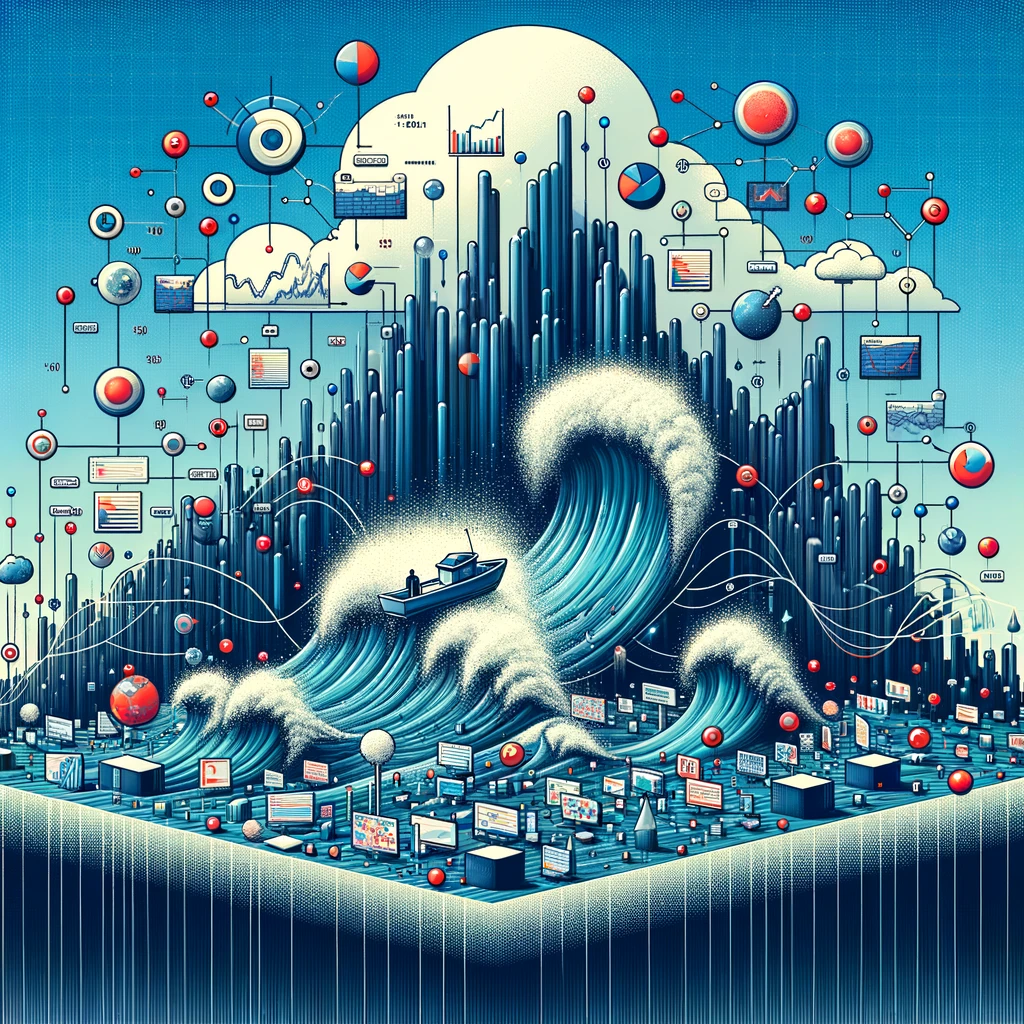
This overreach typically manifests itself in projects that are broad in scope but ambiguous in tactical impact, diverting valuable resources towards endless debates over semantic nuances rather than focusing on actionable outcomes. Such endeavors can cause significant delays and, more critically, lead to the abandonment of the core objective: delivering practical value to the organization. By striving to capture every possible data relationship and nuance, teams risk losing sight of the model’s intended purpose—to serve as a functional tool that supports real-world decision-making and operations.
The consequence is a sprawling, often incomplete model that, while theoretically robust, fails to align with the strategic and operational needs of the business. Furthermore, this approach can often lead to models that introduce excessive complexity at query time. A seemingly straightforward question like, “How many clinical trial participants had complications this calendar year?” can unravel into a convoluted mess when trying to extract answers from the graph.
The challenge, then, is not just to avoid this exhaustive approach but to define a more targeted, pragmatic strategy to drive meaningful results and enhance organizational performance.
Advantages of Bite-Sized Knowledge Modeling
We suggest an approach that mirrors the well-established principles of Agile software engineering, championing a controlled but rapid, incremental delivery of value. By focusing on delivering smaller, manageable segments of a model that directly address specific business needs, organizations can achieve quicker wins and continuous improvement. This adaptive approach not only aligns with the dynamic nature of business environments but also allows for flexibility in response to evolving requirements and insights. This way, each incremental improvement builds upon the last, steadily enhancing the overall utility of the semantic model while avoiding the overwhelming complexity that often stifles progress in more monolithic projects.
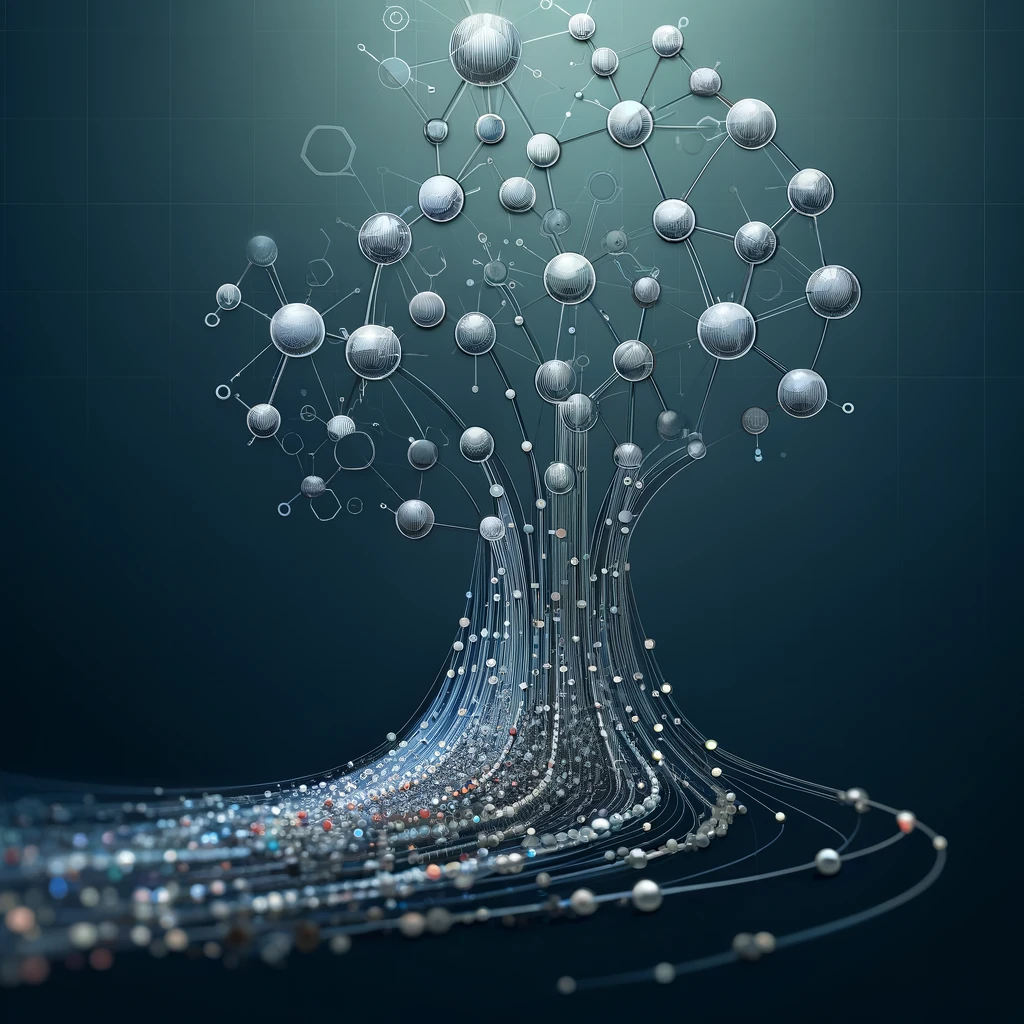
Velocity Made Good on Course (VMC)
In the realm of navigation, the concept of ‘velocity made good on course’ (VMC) is crucial for efficiently reaching a destination. It’s not just about speed, but speed in the right direction. Similarly, in the realm of knowledge modeling, the bite-sized approach aligns perfectly with the principle of VMC. By focusing on small, manageable projects that directly address specific business needs, organizations can ensure that every step taken is effectively moving them closer to their return on investment (ROI). This targeted approach minimizes deviations and avoids the pitfalls of expansive modeling projects that often meander far from their intended course. Just as a navigator adjusts the sails to maintain the best course towards their destination, companies can steer their projects with precision, ensuring that each incremental effort contributes optimally towards the overarching goal of enhanced business value and insight.
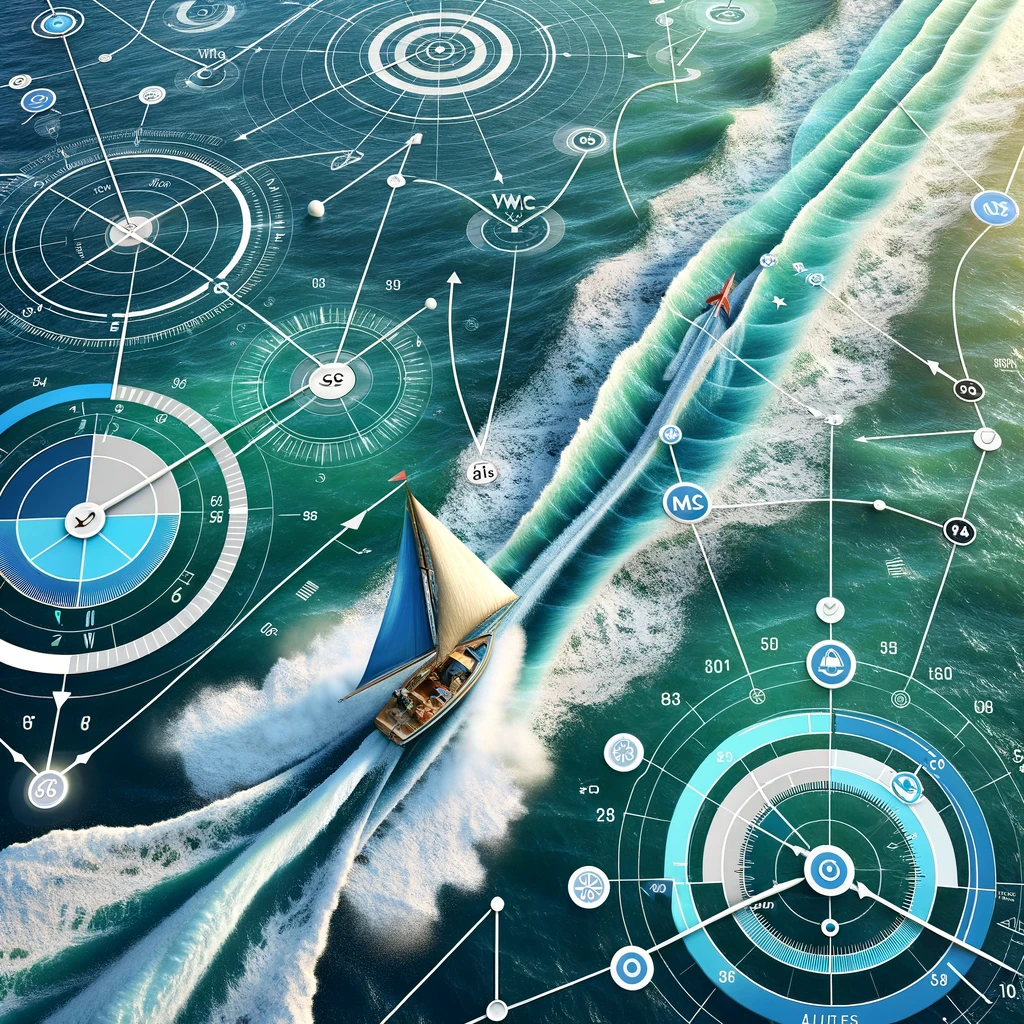
Too Many Cooks? Slice the Kitchen into Culinary Teams
A common adage warns against having “too many cooks in the kitchen,” as it can lead to confusion and a disjointed meal. Similarly, in the vast ecosystem of business, breaking down the larger framework into smaller, more focused sub-domains will significantly enhance efficiency and consensus that will drive better knowledge modeling outcomes. By organizing smaller groups of business experts who share a similar vernacular and expertise, akin to specialized culinary teams working in a large kitchen, modeling efforts can foster a more harmonious environment. Each team, like a group of chefs specializing in appetizers, main courses, or desserts, can focus on modeling their specific area of expertise and driving value where it is more precisely needed. This specialization allows for quicker decision-making and a higher likelihood of agreement, as each group operates within a well-defined scope and with clear objectives.
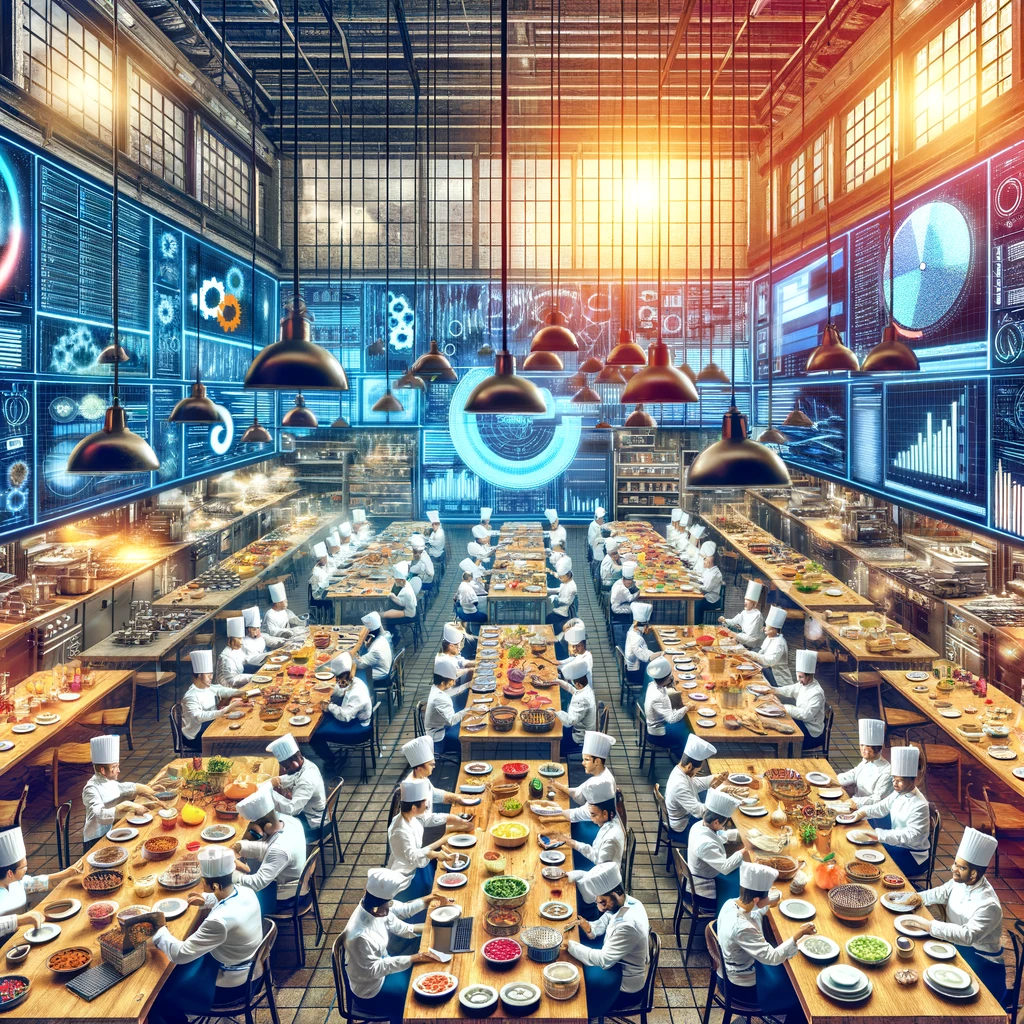
Just as chefs in smaller teams can rapidly adjust recipes, experiment with new ingredients, and refine their dishes based on immediate feedback, so too can smaller knowledge modeling teams adapt and innovate within their domains. This culinary-inspired approach to business organization not only streamlines progress but also enhances the overall coherence and effectiveness of the company’s strategy, serving up success one well-managed team at a time.
Building Blocks: Laying Foundations w/Bite Sized Ontologies
In this complex world of data and information management, the challenge often lies not just in the volume of data but in making sense of it quickly and effectively. At RealmOne, we’ve honed a pragmatic approach we call “bite-sized knowledge modeling,” which simplifies the process of building semantic models by breaking it down into manageable, clearly defined stages. This method ensures that projects remain focused and deliver tangible value without getting bogged down by the enormity of the data.
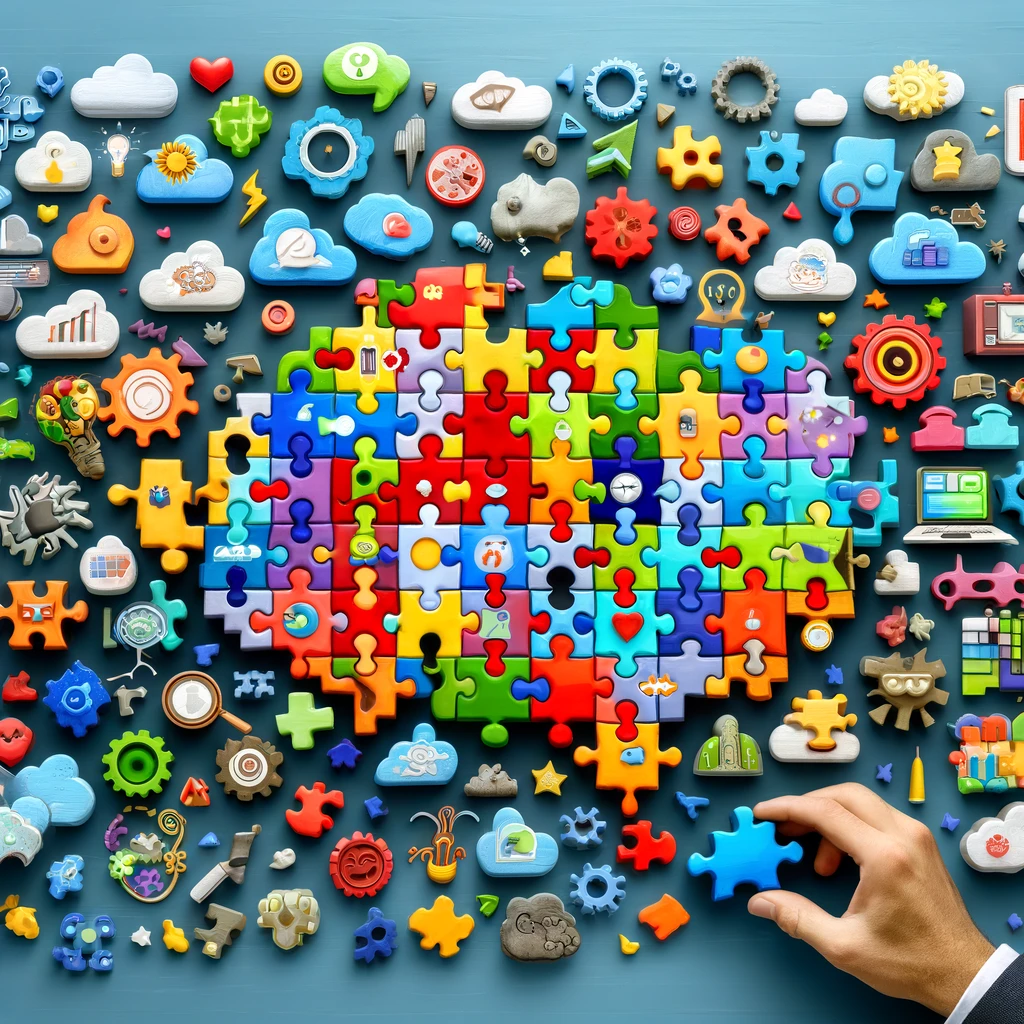
Our suggested approach to semantic modeling leverages the targeted development of small individual ontologies, each finely tuned to address specific domains or use cases. This methodology allows us to focus intensely on the unique requirements of each domain, ensuring that the ontologies are both precise and practical. As these ontologies are gradually layered together, they form a comprehensive tapestry that provides a broader perspective and an integrated solution. Over time, this scalable system not only grows in richness and detail but also in utility, adapting and expanding as organizational needs evolve. This process of layering small, domain-specific ontologies enables us to build out robust solutions that are inherently flexible, allowing for incremental enhancements without disrupting existing data structures.
General Modeling Methodology
In broad practice, we see two general schools of thought regarding semantic modeling. The top-down approach starts with the high-level, philosophical concepts, striving to model an entire domain comprehensively. While ambitious, this method often equates to trying to ‘boil the ocean’— with all the issues we have delved into so far. Conversely, the bottom-up approach begins with the existing physical data models from existing data sources, aiming to build ontologies based on tangible elements. However, this method will lead to models that are too narrowly focused, limiting their potential for reuse and scaling. Furthermore, it perpetuates the ‘stove-pipe’ problem, where lack of semantic consistency across terms leads to significant harmonization challenges.
At RealmOne, we advocate for a bite-sized, middle-out approach, which integrates the strengths of both methodologies while mitigating their weaknesses. This strategy begins by narrowing the expansive vision of the top-down approach to focus more directly on practical applications and identifiable business value. By starting from a defined scope that addresses specific organizational needs, we deliberately avoid the pitfalls of overly abstract conceptualizations – please note that it takes conscious and continued effort from all involved to avoid these traps.
Simultaneously, our approach avoids the limitations of the bottom-up strategy by not strictly confining our models to the constraints of the existing physical data model. This flexibility allows us to construct models that are both adaptable and scalable, enhancing data interoperability across different systems and domains. By leveraging the robust frameworks and design patterns of upper-level ontologies such as the Common Core Ontologies (CCO), our models inherit a structured foundation that supports interoperability and utilizes common design patterns, facilitating quicker integration and more effective solutions.
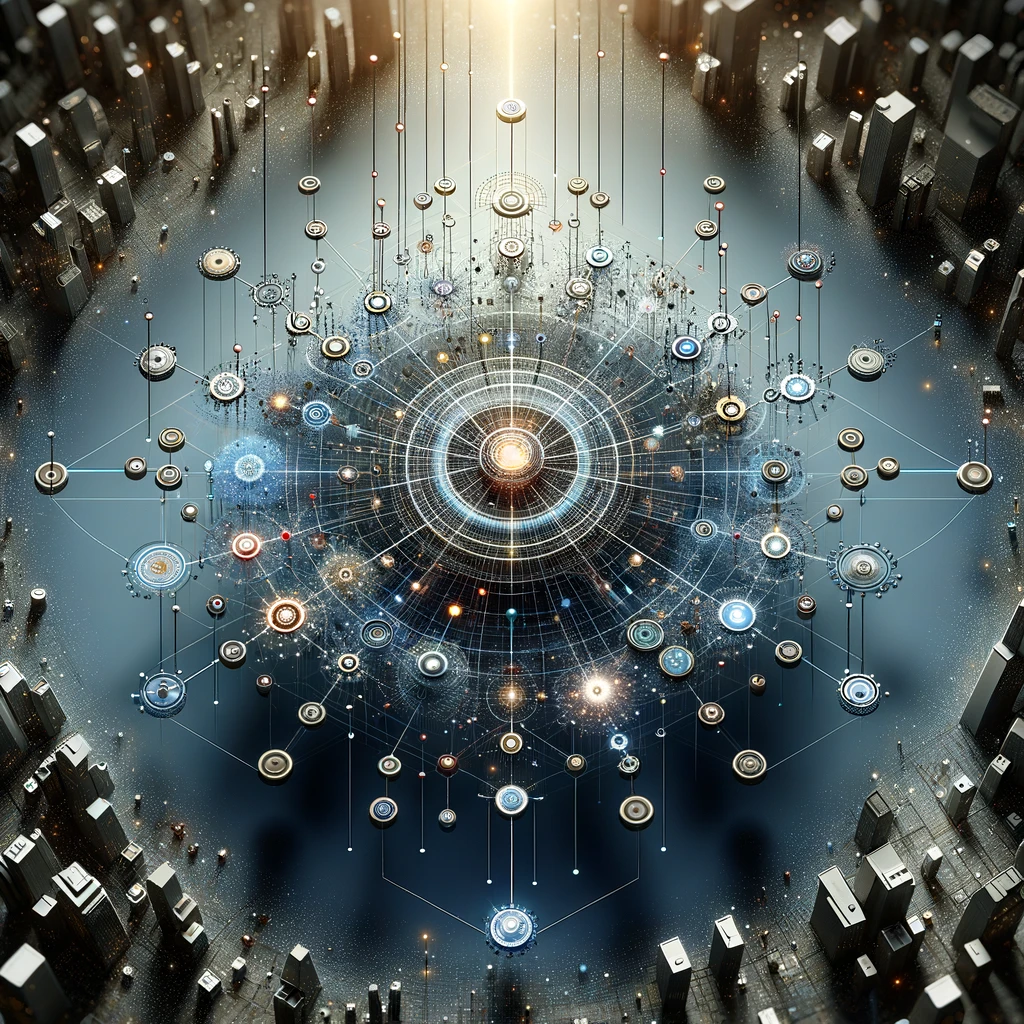
Through this balanced, middle-out approach, we harness the comprehensive benefits of semantic modeling to deliver real, scalable solutions swiftly – transforming ambitious data concepts into practical tools that drive organizational success.
Bringing it Together: What it Looks Like in Practice
- Defining Scope and Competency Questions: Our journey begins with a thorough documentation of requirements and a clear definition of the project’s scope. We frame these requirements as “competency questions” (CQs), which are directly tied to user personas. This step ensures that the end solution is precisely aligned with the needs of its users, addressing the specific questions they need answered.
- Enumerating Key Terms and Pseudo Queries: Analyzing these competency questions helps us identify crucial terms and the relationships within them. From these insights, we craft “pseudo-queries,” which guide the subsequent stages of model development, ensuring that our efforts are rooted in actual user inquiries and needs.
- Researching Existing Ontologies: Instead of starting from scratch, we explore existing ontologies within the target domain. This stage leverages the interoperability of semantic models to accelerate solution development, drawing on established resources to provide quicker value.
- Developing Solution Models: With key terms and external models identified, we define the types, attributes, and relationships within our graph. Decisions made during this stage determine the structure of the data and its representation, always with an eye towards scalability and future growth.
- Selecting Controlled Vocabularies: In parallel, we identify potential controlled vocabularies—preset lists of values that standardize definitions within the graph. This is crucial for enhancing query performance and data discovery.
- Validating Data with SHACL Shapes: Finally, we develop SHACL shapes for validating the graph data, ensuring that the semantic solution adheres to high standards of data quality from the outset.
Let’s Collaborate!
Think these methodologies can be improved or adapted for your projects? We’re always open to ideas and collaboration. Contact Us to discuss more.
Mobi, Obviously…
Sure, we might be a tad biased, but when it comes to a tool to manage the semantic artifacts you develop, Mobi is uniquely positioned to augment and power the methodologies laid out here. Embrace change management without the drama and integrate continuously without breaking a sweat. With Mobi, the complex dance of developing, testing, and deploying semantic artifacts becomes less of a tango and more of a well-orchestrated ballet. Have the various teams in your organization leverage Mobi to collaboratively weave a mosaic of knowledge into practical insight that drives real business value! Download Mobi and Contact Us to give it a try!